Project Reporting in the Age of AI
Robin Steward
|
4 min read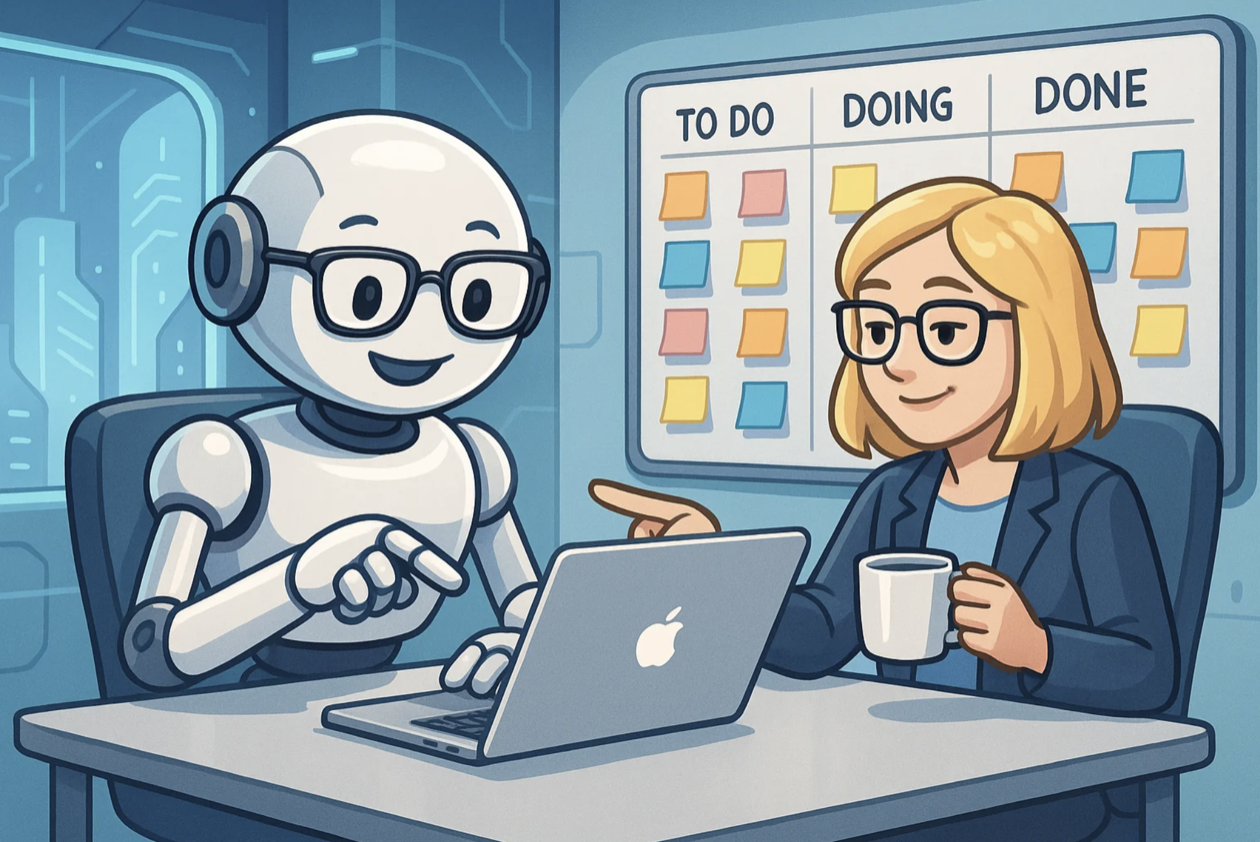
In the world of program and project management, reporting is both essential and often exasperating. Done right, it provides a clear view into progress, issues, and risks, enabling quick decision-making. Done poorly, it becomes background noise — a blur of dashboards, metrics, and slide decks that obscure more than they reveal.
At a time when systems are becoming more complex, delivery cycles are accelerating, and executive expectations are higher than ever, stakeholders want more than just raw status details and metrics. They want clarity and insight, and they want it quickly.
In response, many organizations are turning to smarter tools — including AI — to simplify reporting and surface what matters most. But while automation and AI can accelerate the process, they don’t replace the human judgment that makes reporting meaningful. Especially in fast-moving Agile environments, clarity depends on a thoughtful balance of data, metrics and story.
The Real Job of Reporting
Reporting isn’t just about artifacts — it’s about alignment. Jira can generate a plethora of charts and reports based on both project and empirical data. But understanding what the metrics mean, and how to communicate them in a way that’s useful to different stakeholders, requires human interpretation. Executive sponsors don’t want a swim lane deep dive or a Jira sprint report — they want to know the project status as well as where the risks are and how we’re mitigating them. Product owners want to know how changing priorities impact near-term velocity and their roadmap goals. Engineers want to know what’s blocking progress, not just what’s behind schedule
AI as a Companion
There has been a shift toward using AI to generate reports, summarize sprint retrospectives, or flag potential delivery issues. Some teams use LLMs to analyze team notes or draw insights from Jira histories. Others rely on built-in analytics or plugins to identify blockers or project drift.
Natural Language Processing (NLP) can extract patterns from unstructured data — such as recurring issues in sprint comments or customer feedback. Predictive analytics can be used to forecast scope creep or resource contention across program increments. Generative AI can draft updates to summarize hundreds of Jira tickets into a few coherent paragraphs.
But these tools alone don’t have enough context to understand why the surfaced insights matter. In fact, research shows a strong negative correlation between how ‘automatable’ a task is and how “messy” the task environment is. When information is scattered across Jira, GitHub, Slack, iCloud, and hallway conversations, LLMs don’t have access to it all, and therefore struggle to correctly infer relevance or priority over what they do have access to. And on top of that, a single hallucinated assumption can derail an entire report.
These tools can accelerate aspects of the job, but cannot be relied on too heavily. All of these tools need context, configuration, and most importantly, interpretation. Left on their own, information could be excluded, nuances could be misrepresented, noise could be elevated, and summaries could be generated that miss the point entirely. This is where good project management can make the difference
The Human Layer Still Matters
Even in the most data-rich environments, the role of a project manager isn’t just to report — it’s to translate. Reporting exists to help stakeholders understand why something happened, what it means, and what’s next.
The best reports are the ones that drive alignment, foster trust, and make the next decision easier — not harder. And achieving that isn’t about adding more data. It’s about telling a clearer story.
Frameworks provide a basic structure and foundation for projects, but humans are needed to make those structures effective. That means knowing your audience, focusing on what matters, and shaping updates into narratives that drive decisions.
Effective project managers are responsible to provide:
- Contextual Framing: A report may show team velocity dropping, but the project manager understands the root cause and is able to convey if there is an issue (e.g. onboarding, unplanned activity) or if project is on-track.
- Audience Tuning: Engineers want actionable backlog updates. Executives high-level status and risk mitigation plans. Tailoring the message is key.
- Synthesis and Focus: With multiple teams and streams running concurrently, surfacing an issue to facilitate quick and accurate decision making requires discernment. The saying “If everything is a priority, then nothing is a priority” holds true.
Smart Reporting Practices
Whether you’re using spreadsheets or AI-driven dashboards, the following guidelines can help elevate reporting from routine status to ones that can help provide strategic value:
Let AI Handle the Repetitive, Humans Handle the Relevance
Use AI to automate data gathering, generate initial summaries, or flag anomalies, but don’t outsource the story. Project managers are needed to add context to turn information into insight.
Translate Data into Decisions
AI can help surface trends (e.g., velocity dips, recurring blockers), but project managers should interpret why it matters. Effective status reports should enable decisions or actions.
Maintain Consistency
Even with AI-generated inputs, ensure there’s a consistent tone and narrative thread. This helps stakeholders trust and connect with the report — especially over time.
Looking Ahead: The Balance of Systems and Storytelling
As delivery environments become more complex and interconnected, the challenge of reporting only grows. Agile frameworks and tools provide foundational structure, and AI gives us speed – but the clarity comes from good project managers who can “see the forest through the trees”.